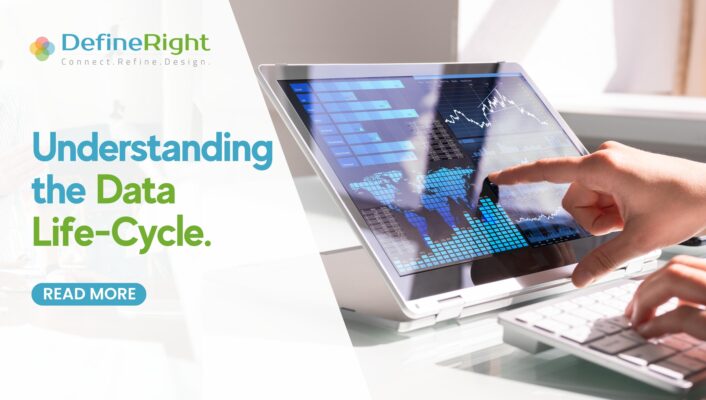
Data is an essential part of any organization, and understanding its lifecycle is crucial for ensuring its integrity, security, and usefulness. The lifecycle of data refers to the stages that data goes through, from its creation to its eventual disposal. While there is no one best way for data classification, different organizations may classify data stages differently based on the quantum of data, the nature of their business, and other factors.
However, for the purpose of this article, we will focus on a framework that consists of five stages. Organizations can keep this framework in mind, add sub-steps or ignore some steps to make it fit their purpose. These stages of the data lifecycle include the following: Creation, Quality & Benchmarking, Processing, Analysis, and Disposal.
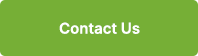
Data Creation – Generation of Data
The first stage of the data lifecycle is creation. This stage involves the generation of data, which can come from a variety of sources. For example, data can be created through customer transactions, sensor readings, social media posts, and more. Data creation can occur in various forms, such as structured, semi-structured, and unstructured. The good thing is that with the advancement in technology, it has become easier to structure even the most complex data sets.
If we talk specifically about Clinical Development Data Systems, data is collected from various sources such as electronic data capture (EDC) systems, electronic medical records (EMR), paper or electronic case report forms (CRF), Interactive Voice Response System (IVRS), and many others. Data collection is typically done in accordance with Good Clinical Practice (GCP) guidelines and the Study’s design protocol and often has a defined way in which a particular trial’s data is collected.
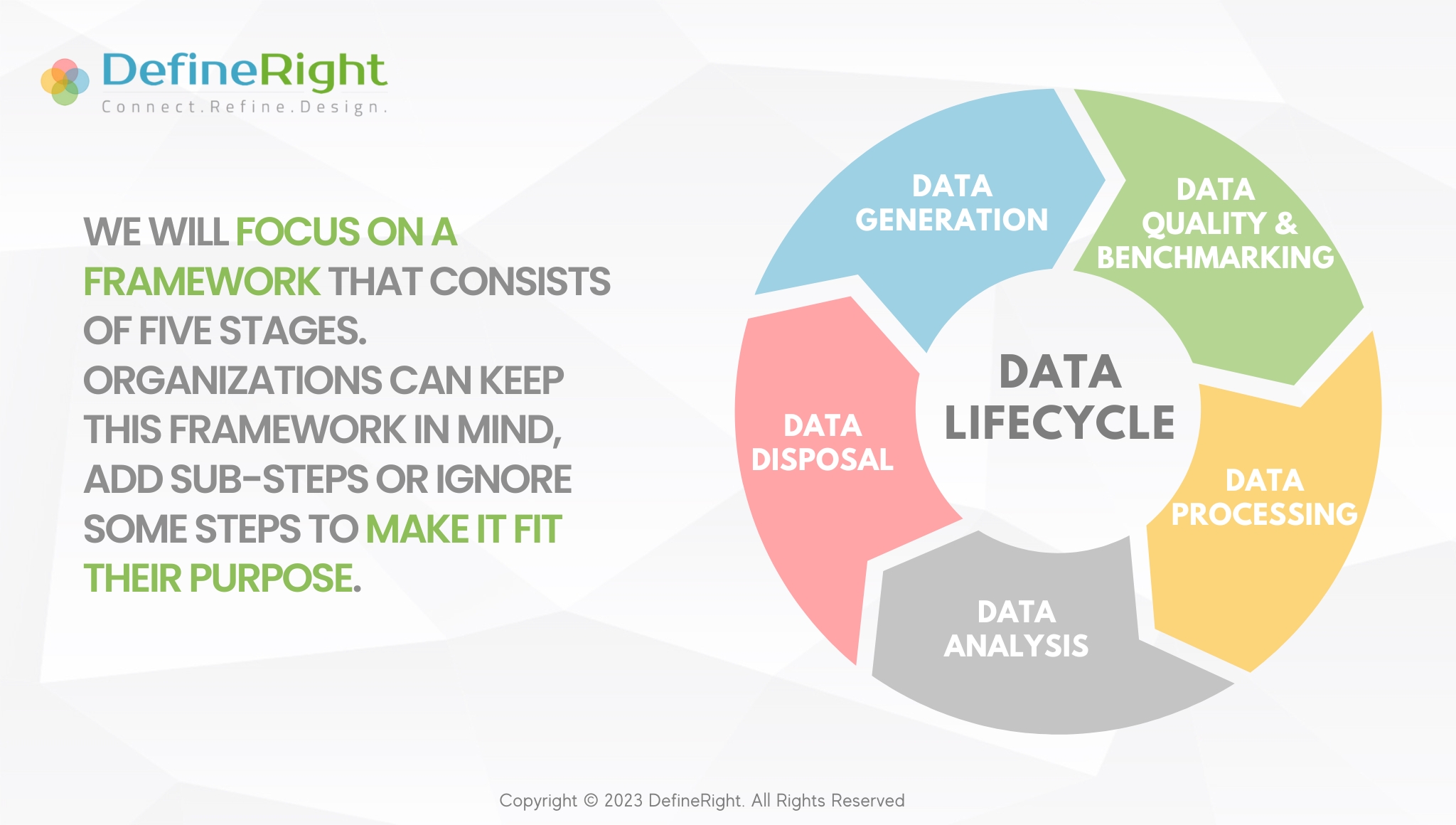
Data Quality and Benchmarking
Once data is created, it moves on to the storage. This is where data is saved in a physical or digital format, such as a database or a file system. Though the storage stage is crucial for ensuring the integrity of the data and making it accessible for further processing and analysis, there needs to be a focus on the quality of data as well.
Benchmarking is also an essential part of the process. More often than not, data comes from multiple sources and disparate systems. Before anything meaningful can be done, it is important to check for data quality and make an effort to clean it at source to ensure that insights at later stages are more meaningful. Data is managed and validated during this stage. This includes tasks such as data verification and quality check via business rules. Data management ensures the quality and integrity of the data.
Data Processing – Transforming Data into Usable Format
The next stage is data processing. This is where the data is transformed and converted to a refined format to make it more usable and accurate. Data processing can include tasks such as data integration, data transformation, data validation, and data warehousing. This stage is important for ensuring that the data is in a format that can be easily analyzed and used for reporting.
Nowadays, certain tools have an inbuilt analytics component that allows the processing and analysis to be done in a single tool. Having said that, it is not the tool but the business problem at hand that determines what is the best strategy for any organization.
Data Analysis – Extracting Insights for Better Decisions
After the data is processed, it moves on to the analysis stage. This is where the data is analyzed to extract insights that can be used to make better decisions. Data analysis can include tasks such as data mining, machine learning, and statistical analysis. This stage is important for uncovering patterns and trends in the data that can be used to improve business processes and operations. Data analysis can include tasks such as descriptive statistics, hypothesis testing, and predictive modeling.
Data Governance and Disposal
Finally, the data reaches the governance stage. As a first step, the efficacy of the complete data processes is measured at a suitable cadence, and improvisations to the process are identified. This is where the data is deleted or archived to free up storage space and reduce the risk of data breaches. Data disposal can include tasks such as data deletion, data archiving, and data anonymization. It is important to have a proper data retention and disposal policy in place to ensure that the data is disposed of in a secure and compliant manner.
Archiving or Storing Data Securely
After the study is completed, the data is archived for future reference. Archiving can include storing the data in a secure repository or converting it to a non-editable format. Data is typically archived in accordance with regulatory guidelines and industry standards. Eventually, the data is disposed of according to a data retention policy. This can include data deletion, anonymization, or destruction of physical records.
Data Protection Is of Utmost Importance in the Data Lifecycle
Throughout the data lifecycle, it is important to ensure that the data is protected and secure. This includes implementing security measures such as encryption, access controls, and backups. Additionally, compliance with laws and regulations such as GDPR and HIPAA must also be taken into consideration.
Expert Data Lifecycle Management for Greater Business Outcomes
In conclusion, the data lifecycle is a continuous process that helps organizations effectively manage their data. Understanding the stages of the data lifecycle and implementing appropriate controls and processes can help organizations ensure the integrity, security, and usefulness of their data. By managing the data lifecycle effectively, organizations can gain valuable insights and make better decisions, ultimately leading to improved business outcomes.